Consistent muzzle velocity is key for long-range shooting, otherwise, bullets that leave the muzzle faster than normal could miss high, or bullets that leave the muzzle slower could miss low. While the goal is for each shot to leave the muzzle at precisely the same velocity, no ammo is perfect. So it is very helpful for us as long-range shooters to understand the variation we can expect from our ammo shot-to-shot.
To see how much consistent muzzle velocity matters in terms of hit probability at long-range, read How Much Does SD Matter?
A primary goal of many long-range handloaders is to develop ammo with optimal muzzle velocity consistency, so this article will be 100% focused on helping us get more insight and make better decisions related to that. It will explain the different methods shooters use to quantify variation in velocity, dispel a few common misconceptions, and provide some practical tips.
Part 1 laid the foundation that we’ll build on here, so if you haven’t read it I’d start there. The next article, Part 3, will focus on the application of similar concepts when it comes to analyzing group size and dispersion.
Extreme Spread (ES) vs. Standard Deviation (SD)
The two most common stats shooters use to quantify variation in muzzle velocity are:
- Extreme Spread (ES): The difference between the slowest and fastest velocities recorded.
- Standard Deviation (SD): A measure of how spread out a set of numbers are. A low SD indicates all our velocities are closer to the average, while a high SD indicates the velocities are spread out over a wider range. (Note: Part 1 explained SD in detail, so please read that if you aren’t familiar with it – or the rest of this won’t make sense.)
Some shooters have strong opinions about which of those two measures are most applicable or relevant when it comes to long-range shooting, and they might completely ignore one or the other. But remember this important point from the last article, when it comes to any descriptive statistic, like ES or SD:
“Descriptive statistics [like ES and SD] are very good at summing up a bunch of data points into a single number. The bad news is that any simplification invites abuse. Descriptive statistics can be like online dating profiles: technically accurate and yet pretty darn misleading! Descriptive statistics exist to simplify, which always implies some loss of detail or nuance. So here is a very important point: An over-reliance on any descriptive statistic can lead to misleading conclusions.” – Charles Wheelan
So it’s probably a bad idea to be completely dismissive of either ES or SD. Both provide some form of insight. There are scenarios where SD is the best to use, and other scenarios where ES may be more helpful. I’ll try to provide a balanced perspective on when we should use one or the other.
In general, SD is usually a more reliable stat when it comes to quantifying the muzzle velocity variation. Adam MacDonald explains an important aspect when it comes to ES: “For normally distributed sample data, the extreme spread is a misleading measure of the variation because it ignores the bulk of the data and focuses entirely on whether extreme events happened to occur in that sample.” (Not sure what “normally distributed” means? Read Part 1.)
The example below shows muzzle velocities recorded over 20 shots, and we can see the ES is entirely dependent on the difference between shot #11 (the fastest) and #17 (the slowest). All of the other 18 shots are ignored when calculating ES. The ES is simply the max (2790 fps) minus the min (2752 fps): 2790 – 2752 = 38 fps.
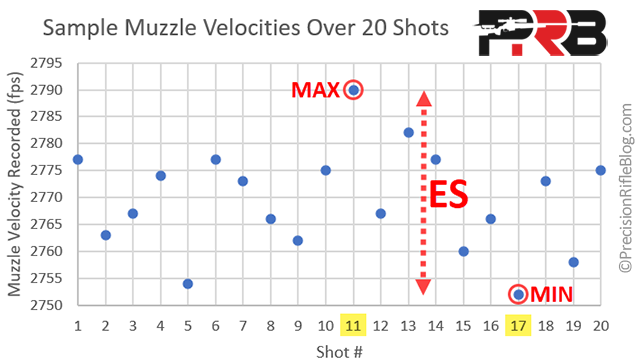
The ES is by definition focused on the two most extreme values. MacDonald suggests, “We should instead be focusing on describing the results that are most likely to happen again. We need a metric which best represents the variation that we care about describing, and takes all the data into account. This is the standard deviation (SD).” He adds another important nuance between SD and ES: “As you collect more and more data, the measured SD of that sample becomes closer and closer to the true SD of the population. In contrast, the extreme spread will always grow with sample size, as more and more extreme events occur over time. It’s easier to measure, but it’s not nearly as reliable as the SD.”
MacDonald is not alone in this view. Most people who are familiar with statistics believe SD is a better statistical indicator of muzzle velocity variation. In fact, Engleman says it this plainly: “ES is not a reliable statistical indicator. The best indicator of velocity variations is the standard deviation.”
If we look back to the example of 20 muzzle velocities shown above, we can see how the ES grows as we fire more and more shots. The chart below shows what the ES was after each of the 20 shots. After the 2nd shot, the max velocity is Shot #1 at 2777 fps and the min velocity is Shot #2 at 2763 fps, so our ES is 14 fps (2777 – 2763). Shots 3 and 4 land between those extremes, so the ES remains the same until Shot #5 registered down at 2754. That is 9 fps lower than our previous minimum, so at that point, our ES jumps up to 23 fps. Then it remains there until Shot #11 at 2790, which is 13 fps faster than our previous max of 2777, so our ES increases to 36 fps. Finally, Shot #17 registers at 2752, which is 2 fps slower than our previous minimum, so our ES grows to 38 fps. The chart below shows the progression of how ES changes over those 20 shots:
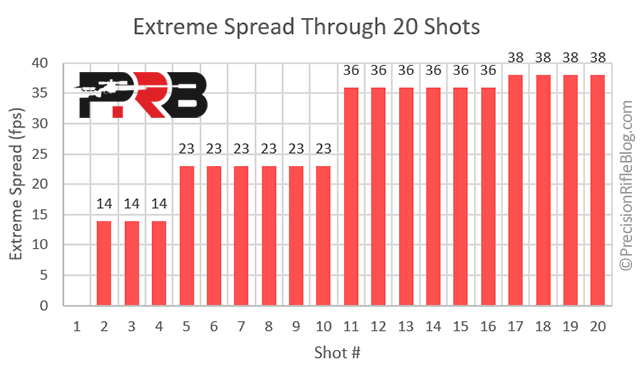
We can see if we’d have only fired 10 shots, we’d leave the range fully convinced that our ammo had an ES of 23 fps. But, when we fired shot #11, that one shot caused the ES to increase by over 50%! It was blind luck that round was Shot #11 and not Shot #2 or #20.
Because ES is ultimately based on two shots, a single shot could increase the ES drastically. However, because the SD is calculated using every data point, the more shots we fire, the less impact any single shot will have on the result. The chart below allows us to see how the SD starts to “stabilize” as the number of shots grows. The more shots fired, the less drastic the SD changes. However, the ES changes more sporadically through the 20 shots and would continue to increase if we continued to fire shots. If we fired 5 more shots, our ES might stay at 38 or go to 50+. However, it’s unlikely our SD would change drastically from what is shown. In fact, based on the 20-shot data we can predict with 90% confidence that even if we fired another 1,000 shots our SD would end up between 7.6 and 13.2 fps.
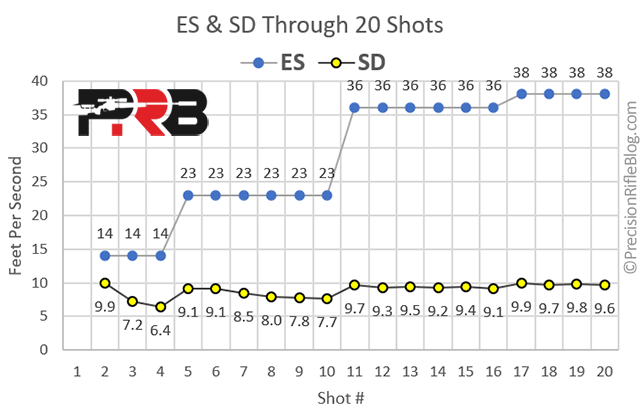
Some shooters believe ES is more applicable because they like to calculate if their shots would still hit a certain size target at their extreme fastest and slowest velocities. If their lowest muzzle velocity they recorded was 2,752 fps and their fastest was 2,790 fps, they could plug each of those into their ballistic calculator and see if the predicted drop would still result in an impact on a coyote-sized target at 600 yards. That seems relevant, right? But, if we have an accurate SD, we can actually use that to make a very good prediction of the true ES – possibly even more indicative of what the population might be than what we measured directly from a sample.
“The true extreme spread of a population is about 6 times the standard deviation,” explains Engleman. That is thanks to the power of a normal distribution, which we talked about in the last article. In a normal distribution, we know 99.9% of our shots will be within 3 SD (3 times the SD) from our average velocity. That means our slowest shot would be 3 times our SD below the average, and the fastest shot would be 3 times our SD above the average – and the difference between the min and the max would be very close to 6 times our SD. So, in our example where our SD is 9.6 fps, we should expect our ES to eventually grow to 58 fps if we continued to fire shots (9.6 fps SD x 6 = 57.6 fps ES). How many shots would it take before measured ES got to 58 fps? Who knows! It might take 100+ rounds, but we also might luck into that spread in a different 20-shot string. But, by 1,000 shots our data would take the shape of a normal distribution and our ES would likely land near 60 fps, yet our SD would very likely remain between 8 and 13 fps. This illustrates why SD is a more reliable statistical indicator of variation.
Is SD perfect? No! Any time we summarize a bunch of data with a single number, we are losing some nuance or detail. But, SD is certainly useful for quantifying muzzle velocity variation. Of course, there are some scenarios when ES might be more helpful, and I’ll try to point those out in this article.
What Is A “Good” SD?
If you’re newer to the concept of SD, you might be wondering, “What is a ‘good’ SD when it comes to muzzle velocity?”
It’s relatively easy for a reloader to produce ammo with an SD of 15 fps, but we typically have to be meticulous and use good components and equipment to wrestle that down into single digits. The table below is from Modern Advancements in Long Range Shooting Volume 2 and it provides a “summary of what kind of SD’s are required to achieve certain long-range shooting goals in general terms”:
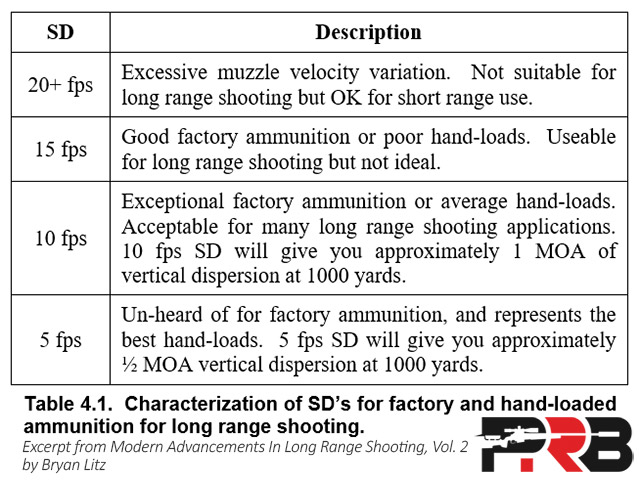
In general, most long-range shooters have a goal to have ammo with SD’s “in the single digits” (i.e. under 10 fps). Those engaging targets beyond 2,000 yards in Extreme Long Range (ELR), where first-round hits are critical, want to be closer to that 5 fps. The lower the better! 😉
For more context, read How Much Does SD Matter?
The Problem With SD: Sample Size Matters – A Lot!
At this point, we’ve established that SD is a superior statistical indicator of muzzle velocity variation, but there is a pitfall when it comes to SD that most shooters aren’t aware of: While it’s easy to get close to the average muzzle velocity with a smaller sample size, “but the SD is a different story. It’s a lot more difficult to measure variation than most people would assume,” explains MacDonald. If we want to have much confidence that our results represent the population we likely need a larger sample size than you think. Denton Bramwell agrees by saying, “Standard deviation is hard to estimate with precision.” Bramwell goes on to say that “changes in standard deviation are devilishly difficult to reliably detect,” and there is a “tendency to underestimate variation [and therefore SD] in small samples.” Engleman corroborates that point, saying, “Testing for velocity variations requires larger sample sizes – 5 shot samples will not yield reliable results.”
Many shooters see SD as an indicator of ammo quality (i.e. the lower the SD the higher the quality), so it’s common to see people brag about their low SD on the internet. They may even post a photo showing the stats from their LabRadar or MagnetoSpeed – and you can see it was over a 5 shot string. 5 shots is simply not a large enough sample size to have confidence in an SD. In fact, let’s say that someone had a 9 fps SD over 5 shots. For a 95% confidence interval, we would predict the real SD over a large sample size would be between 5.4 and 25.9 fps! The truth is, we can’t have much confidence in knowing our SD without a larger sample size. Many people record 10-shot strings, but the more the better! If we’re just trying to determine the average velocity, a 10-shot string is likely adequate, but when trying to quantify variation within a batch of ammo, we may need to record a string of 20 shots or more.
Engleman conducted a very interesting study where he recorded velocities over 50 consecutive shots, and then went back and grouped those results into distinct 5, 10, and 20 shot strings. He explained, “We will investigate scientifically sound methods of using 5, 10, or 20 shot strings to characterize a load combination. To do this, artificial strings will be chosen from the 50 shots already fired.” For example, forty-five 5 shot strings can be produced as follows:
- String 1: Shots # 1, 2, 3, 4, 5
- String 2: Shots # 2, 3, 4, 5, 6
- …
- String 45: Shots # 46, 47, 48, 49, 50
Note that we aren’t changing the order in which any shots were fired. Each of the strings includes shots captured in exact sequential order. If we only fired 5 shots from among the 50 recorded, it could have easily been any one of these artificial sets of sequential shots. Engleman followed the same method to create forty 10-shot strings and thirty 20-shot strings from the 50 sequential shots recorded.
His results show how much the average, SD, and ES could have varied based on the luck of the draw in terms of what shots happened to be included in each string:
Here is an important thing to notice: Think about if we are doing load development, and we’re trying to decide between two loads based on the SD of a 5-shot string we fired of each. This example shows that if we only fire a 5-shot string there is a chance we might measure an SD of 5 fps or 14 fps from the exact same batch of ammo! We’d obviously pick the load that produced the lower SD and might believe we had stumbled on a great load that is “tuned” for our rifle, although the lower SD was actually due to complete random chance and well within the natural variation we should expect from such a small sample size.
In Engleman’s results above, we can see the red lines change the most, which is the line indicating the possible output from a random set of 5 sequential shots. We can see on the top graph, depending on what 5 rounds we pulled out of the box, the average muzzle velocity might measure anywhere from 2982 fps to 2996 fps. Here is a summary of the ranges for each metric based on the number of shots in each group:
# of Shots In String | Average MV | SD | ES |
5 Shots | 2982-2996 | 4-14 | 12-40 |
10 Shots | 2985-2992 | 7-12 | 20-40 |
20 Shots | 2987-2989 | 8-11 | 30-45 |
Notice for all 3 metrics (average, SD, and ES), the more shots in each string the narrower the range of possible outcomes became. Simply put the more rounds we fire, the more certain we can be of the results. But, we can also see in the table above that as we fired more shots both the average and SD began to converge, but ES had a different pattern. The more rounds fired the higher the range of possible ES’s went. We shouldn’t be surprised that the highest ES occurred in the 20-shot string. That is part of the downside of ES: We should expect it to grow with sample size, where average and SD will begin to converge on the true value and don’t simply continue to grow the more shots we fire.
Here is Engleman’s key takeaway from his research:
“The first and most important fact presented in this paper is that random 5, 10, or 20 shot sequences all result in different statistics than the ones calculated for all 50 shots. In statistics, we refer to these smaller sequences as samples of the 50 shot population and the statistics generated by the samples are referred to as estimates of the population statistics. Thus, if during the winter off season I load 1000 rounds for competition, I may go to the range in the spring to estimate the expected performance of the total 1000 round population by randomly selecting 5, 10 or 20 rounds to test. I am not interested in the statistics of this sample shot sequence so much as we are interested in estimating the performance of the entire 1000 rounds. As can be seen from the data presented in Figure 3, it is very unlikely that the statistics from this sample will be exactly that of the 1000. To be clear, if you fire 5 shots from the 1000 you made over the winter over your chronograph and it tells you the SD = 4, then the standard deviation calculated for those 5 shots is exactly 4. But the standard deviation of all 1000 rounds is unlikely to be 4. The number presented on the chronograph is just an estimate of the total performance and the chronograph will not tell you how good a ‘guess’ it is.”
For SD in particular, Engleman went further and calculated the “90% Confidence Intervals” based on the number of shots in each string, and charted those along with the output for his “hypothetical strings” (if you aren’t sure what “confidence intervals” are, read Part 1):
We can see the range is the largest for the SD that is based on just 5 shots, and the range is much more narrow for the SD based on 10 shots and even tighter for the SD based on 20 shots. Engleman points out, “It is interesting to note that Sample #16 from the 5 shot samples is actually below the bound. 90% confidence means 1 in 10 chances may fail. String #16 was a very lucky string, but it was the only one of 45 possible strings outside the bounds which is much better than 90% success rate. Want better than 90% certainty? Either we have to make the confidence intervals wider or use more shots in the sample. There is no free lunch here – estimating standard deviations from samples is hard work.”
So, what is a good sample size? The answer depends on how minor the differences are that we’re trying to detect and how much confidence we want to have in the results being predictive of the future. When I am trying to quantify the variation in muzzle velocity for my ammo, I typically fire a minimum of 20 sequential shots. More recently, I’ve started taking my LabRadar with me when I practice long-range. Instead of only using it to record an occasional string of shots when I’m checking my zero or doing load development, I set it up and keep it running while I’m practicing from prone and I might record 30+ shots in a single range session. It doesn’t require me to fire any more rounds than I would have otherwise, but I’m simply gaining more value and insight about the true performance of my ammo.
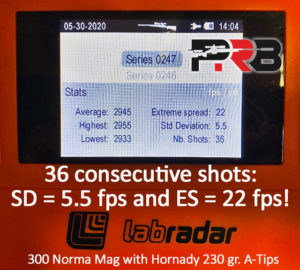
During load development, we should also be cautious to draw conclusions from differences in SD’s that are a result of less than 20 shots. Even if we fired 10 shots of two different loads, and Load A had an SD of 8.3 fps and Load B had an SD of 11.5 fps – real statistics would tell us those are too close to know if one is truly superior to the other.
Bramwell explains, “As a rule of thumb, 22 data in each group is needed to detect a 1:2 ratio between two standard deviations, about 35 data in each group are required for a 2:3 ratio, and about 50 in each group are required for a 3:4 ratio. In a practical example, if you think you have lowered the standard deviation of your muzzle velocity from 20 to 15 fps, you’ll need to chronograph 100 rounds, 50 from each batch. If the ratio of the two standard deviations comes out 3:4 or greater, you’re justified in saying the change is real.”
As I mentioned in the last article, Adam MacDonald created an extremely helpful online calculator where we can enter our velocities for two loads and it will tell us how much confidence we can have that there is a true performance difference between the samples or if there is so much overlap it is more likely due to natural variation for the sample size we used. No crazy math or talk about F-Tests and P-Tests. Adam made it simple for us to input our data in plain English and get the results in plain English. Here is a link to Adam’s Stats Calculator for Shooters (alternate link).
It is very difficult to determine minor differences in variation between two loads without a ridiculously large sample size (like 100+ rounds each). Bramwell sums it up with this:
“Given the twin burdens of large sample size and sensitivity to non-normality, it is much more difficult than most people expect to tell if a change in standard deviation is real, or if it was just our lucky day. Consequently, a lot of people perform tests, and draw conclusions on truly insufficient data.”
I’ve heard a respected researcher say he usually only pays attention to differences of 15% or more, and even when his team finds those, they’ll fire more rounds to confirm what they’re seeing is real and not simply random variation from a smaller sample size.
Correcting For Smaller Sample Sizes
I realize most of us aren’t going to fire 100 or even 30 round samples for each powder charge and seating depth we try in our load development. So is there something we can do to make better decisions when working with 3 to 10 shot groups?
When we have very small sample sizes, we must realize that variation is typically under-estimated. In fact, when a sample has less than 30 observations, statisticians often apply correction factors to adjust for that.
“When we measure the outcome of a random process just a few times, we tend to underestimate the true variation. That is, if we continue to measure the outcome, the standard deviation of the (increasing) sample size tends to increase. This is very important for shooters because we typically judge performance based on 3-10 shot groups,” explains Bruce Winker. “Range data must be corrected for this effect. … To get a more accurate estimate of the standard deviation of the actual distribution, we will need to apply a correction factor to the group size and muzzle velocity data that we measure at the range.” Bruce explains more about how that works in A User’s Guide To Dispersion Analysis.
Instead of applying a correction factor, Bramwell suggests another approach: “For samples as small as five or so, use range [e.g. ES] instead of standard deviation.” Very small sample sizes are one of those niche scenarios where ES can be a useful stat.
ES can help us eliminate or rule-out loads early on. This is based on the principle that over the long haul we can expect our ES to be around 6 times our SD, right? So, if our measured ES is more than 6 times whatever our SD goal is, we know that load isn’t going to get us there.
Adam MacDonald agrees with Bramwell, sharing his rule of thumb: “Use extreme spread to rule out a bad load with 5 shots. Use standard deviation to prove a good load with confidence.”
Let’s say we are developing a load for Extreme Long Range competitions and our goal is to find a load that produces an SD around 7 fps or less. If our goal for SD is 7 fps, then we expect our ES will be 42 fps (7 x 6 = 42). If after a few shots with a certain load our ES is already 50 fps or more, it’s a safe bet that load isn’t going to get us there and we can move on. Because we expect ES to grow as our sample size grows, we’d likely want to see an ES closer to 4 times the SD over smaller sample sizes (7 x 4 = 28 fps).
How Good Is Your Chronograph?
The final tip I’ll mention about quantifying the variation in muzzle velocity is to understand how precise your chronograph is. Most cheap, light-based chronographs aren’t very precise, and they will add “noise” to your data. If we’re trying to measure SD’s for our ammo into single digits, but our chronograph has 10 fps of SD in its readings – we have a problem! Bryan Litz did a very helpful test of consumer-grade chronographs several years ago, and his results were published in detail in Modern Advancements for Long Range Shooting Volume 1, and I noticed AB also published the full chapter featuring this test online. The chart below shows a summary of the amount of error Bryan measured:
We can see the Oehler 35P produced the most impressive results, with an SD around 1 fps in the equipment readings. The popular MagnetoSpeed had an SD of 3-4 fps. The Shooting Chrony (discontinued) had an SD around 3 fps, but the average it reported was off by 18-22 fps – so I’d call that precise (repeatable), but not accurate (far from the correct value). Beyond those, it quickly turns into a mess. The PVM-21 and SuperChrono were just disasters, in terms of precision and accuracy.
The very popular LabRadar Doppler Radar was released after the Applied Ballistics team did this test. While many people are big fans of the LabRadar, I’m unaware of an objective, third-party test that has been done with the same level of rigor as the Applied Ballistics study. The manufacturer does claim the “LabRadar has an accuracy of 0.1%.” For a reading of 3,000 fps that would be an accuracy of +/- 3 fps, which seems to be comparable with the better chronographs in the Applied Ballistics tests. The anecdotal comparisons (like this one published on AccurateShooter.com, or this comparison against a professional Weibel Doppler Radar) appear to indicate it has similar accuracy and precision to the Oehler 35P and MagnetoSpeed.
If there is noise being added by the measurement device, one way proven way to separate the signal from the noise is to increase the sample size. No surprise at this point! If we want more confidence in the data, the proven path is to simply collect more of it!
Summary & Key Points
We covered a lot of ground, so let’s review some of the key points from this article:
- It’s probably a bad idea to be completely dismissive of either ES or SD. Both provide some form of insight. An over-reliance on any descriptive statistic can lead to misleading conclusions.
- SD is a more reliable and effective stat when it comes to quantifying muzzle velocity variations. ES is easier to measure but is a weaker statistical indicator in general because it is entirely based on the two most extreme events.
- ES will grow with sample size, but average and SD will begin to converge on the true value and don’t simply continue to grow the more shots we fire.
- While it’s easy to get close to the average muzzle velocity with 10 shots or less, it’s exceedingly more difficult to measure variation and SD with precision. There is a tendency for SD to be understated in small samples. To have much confidence that our SD is accurate, we need a larger sample size than many would think – likely 20-30 shots or more. The more the better!
- It is very difficult to determine minor differences in velocity variation between two loads without a ridiculously large sample size (like 50+ rounds or more of each load to differentiate even a 20% difference). Often we make decisions based on truly insufficient data because the measured performance difference between two loads is simply a result of the natural variation we can expect in small sample sizes.
- ES can help to eliminate bad loads early but use SD to prove a good load with confidence.
Other Articles In This Series
Stay tuned for the final article in this series, where we’ll turn our focus to group size and how we can get the most out of the groups we fire and leverage those to make more informed decisions and get more rounds on target.
- How To Predict The Future: Fundamentals of statistics for shooters
- Quantifying Muzzle Velocity Consistency: Gaining insight to minimize our shot-to-shot variation in velocity (this article)
- Quantifying Group Dispersion: Making better decisions when it comes to precision and how small our groups are
- Executive Summary: This article recaps the key points from all 3 articles in a brief bullet-point list
You can also view my full list of works cited if you’re interested in diving deeper into any of these topics.
A clinical pathologist friend of mine actually published an article some time ago on dangers of misinterpreting statistics https://journals.sagepub.com/doi/pdf/10.1177/019262339702500617. He paraphrased the famous quote “Lies, damn lies, and statistics” as once said by Mark Twain. Always remember your outcomes are only as good as your data and the interpretation of said data. In your specific example the biggest risk is confirmation bias. I try and do what you have done and regularly re-chrono my loads to see if what I’m doing remains consistent over time. I think regularly doing this is probably the simplest way make sure you aren’t wasting precious material, time and missing targets.
That’s a great point, Eric. I do think confirmation bias is a huge problem, especially in the shooting community or really any area where someone is attempting to do tests and they aren’t familiar with rigorous research techniques or aware of how deep-seated some of our biases really are. For those who many not be familiar with what that is, here is a simple definition:
Even when our intentions are pure and we really are just seeking the truth, it’s funny how our preconceived ideas of what performs best or what the results will be can creep in. If you aren’t conscious of that and actively thinking about how to mitigate that bias, I’d suspect it will skew your results more than you think.
Keith and Paul left some comments on the last post about randomized testing and even double-blind testing (where both the tester and researcher aren’t aware of which items are which until after the results are collected). If you’re really in search of the truth, those are good ways to reduce bias.
And things change over time. Just think about just your brass. If you are reloading the same brass over-and-over, then it is likely changing slightly each time. It may not be noticeable or a measurable difference between any single firings, but over time I’d suspect those slight changes stack in a way that the performance could be measurably different over a few loadings. Some guys develop a load with new brass or once-fired brass and just assume it will perform like it did in their load development as long as they keep loading it with the same components and powder charge. But even then, your barrel is wearing with every shot – and there is another variable in the equation that is changing underneath us and causing the muzzle velocity and barrel time to change slightly over time. Then you have lot-to-lot variation in powders, primers, and bullets! If you think about rifle performance as an equation or algorithm there are a TON of independent and interdependent variables that influence performance.
In fact, a close friend literally texted me yesterday asking some questions about a rimfire precision rifle and ammo because his rifle was grouping in one hole at 100 yards the last several times he had it out – but that was several months ago and now it’s not. Stuff like that can drive a man insane! Bryan Litz and I had an in-depth conversation about something similar with big bore ELR rifles. Some of those big cartridges are much harder to manage than others. If you are going to shoot it all the time, that is one thing. But if you are going to put it in the safe for a few months and then get it back out to take to a match … some cartridges will perform like they did the last time you shot, and it seems some of them won’t and they require that you fine-tune a load a little each time. (That’s why I went with a standard 375 CheyTac, because it seems less finicky than some of the other wildcats and hotrods.) That’s just the truth of the precision rifle game. It’s part of the game we have to play, at least if your goal is to be competitive at the highest levels or just have a rifle that performs to its full potential.
Thanks for sharing!
Cal
Cal – Great info. I have always been stuck on the ES number but now realize that really does just quantify outliers.
The more basic question I am almost embarrassed to ask but for truing a load what is your process? After you have established velocity node and jump that you like?
Mine has been:
Start with vel from chrono – I have magnetospeed – then true/establish vel at 600-700 yds.
Then stay with that velocity and play with bc to true that up from 800 yds on out?
When does BC have measurable effect, ie at what distance should it be trued? I believe AB wants to true at transonic?
I find my self “chasing” both vel and bc to get things to line up. Can get confusing & frustrating 🤷♂️
Hey, Forest. I’m glad to hear this article has led to some new insight for you. You aren’t alone. I’d bet the majority of shooters rely more heavily on ES. Some of that is just because we’re more familiar with it because that’s how we talk about group size and precision, so we just apply that to velocity too. I appreciate you sharing that this helped you.
For your question about the process for truing your ballistics for a particular load, I could probably write a 3-part series of articles on that question alone! But, I’ll try to give you a brief answer (not necessarily my strong suit 😉 ).
For those who aren’t familiar with “truing” ballistics that basically just means you are tweaking your ballistic engine so that its predicted adjustments better align with where your actual impacts are in the field. My process for that has changed several times over the past decade because the tools have changed dramatically. A couple of years ago I might spend a few hours at the range trying to true my ballistic solution, and even then I might return home with my “truth data” about what my actual adjustments were for the specific distances and atmospheric conditions and play around with an Applied Ballistics Analytics software suite for a couple of hours tweaking my muzzle velocity and BC to best fit the actual impacts I experienced. There were all kinds of tricks to that, and it was a tedious process.
Today my method is much more simple. This year, I’ve been using Hornady’s 4DOF engine and their 4DOF drag curves that are based on the average of Doppler drag files for that particular bullet fired from a variety of rifles. In my experience so far, that typically aligns fairly well with my actual impacts in the field, even before I do any truing. So I don’t use traditional BC’s anymore. If you’re only familiar with BC’s, I’d recommend you read this article: G1 BC vs G7 BC vs Bullet-Specific Drag Models
I am typically running that engine from a Kestrel with Hornady 4DOF, but the free Hornady phone app is based on the same engine. Truing on the Hornady 4DOF is super-simple. I input what my real muzzle velocity is (based on 20 shots over my LabRadar), and just tweak the Axial Form Factor up or down slightly to make it match the actual adjustment was to center shots on a target that is near the end of my supersonic range (i.e. where the bullet has slowed down to around 1300 fps). For many of the mid-sized cartridges I shoot that might be 1400-1600 yards, but for magnums, it is probably a little further. The Axial Form Factor starts at 1.00 by default, and in my experience, I might have to change that to 1.02 or 1.04. I think the most I’ve ever had to adjust it to match my impacts was 1.05. I’d say it’s rare for me to need to tweak it more than 2-4% from the default. After I do that, I am usually always within 1 click of elevation at every distance all the way out to transonic range – which is pretty impressive for how little work goes into that.
If you’re interested in learning more about what the Axial Form Factor and “truing” is actually accounting for, and why every rifle is a little different, you should check out the article I wrote at the link below. I actually think that is one of the most interesting and exciting developments I’ve written about in the past couple of years.
Personalized Drag Models: The Final Frontier in Ballistics
The only other thing I’d say about truing your ballistics is it is incredibly important to get all of the inputs perfect before you start. You can’t guess at things like scope height, twist rate, muzzle velocity, or even atmospherics like exact wind speed and direction and air pressure. Any time you are doing a calibration exercise (which is what truing is), you have to make sure all of your inputs to your ballistic engine are as accurate as possible. It’s also important to have supreme confidence in the distance to your target, your rifle’s zero, and your scope’s click adjustments, and whether the scope is mounted in a way that it tracks perfectly plumb. I would say all the things I just mentioned are things I’ve seen my friends get tripped up on. You don’t have to be OCD about all that stuff except when you are doing a calibration exercise like truing your ballistics … and then you need to be super-OCD about it.
I totally know what you’re talking about regarding chasing your tail as you tweak muzzle velocity and BC. I’d say to stop doing that and start using bullet-specific drag models. Applied Ballistics engines also have similar Doppler based drag files that they call Custom Drag Curves that work similarly to the Hornady 4DOF curves. I don’t think the Applied Ballistics engine is as easy to true as the Hornady engine, and I’ve personally given them that feedback. They have something called Drop Scale Factor that trues based on mach speed, which I can understand the technical merit of that approach – but it is not as straight-forward from an end user’s perspective. Sometimes it automatically overwrites previous data that was stored when you add additional points in the DSF, and that can produce some unexpected results. The Hornady method of just tweaking the Axial Form Factor slightly up or down like you would a BC is very easy and more user friendly and based on my experience it is extremely accurate, too.
Certainly not a brief answer, but I hope this helps! 😉
Thanks,
Cal
Most Excellent!!! I like where you are taking this. I know it takes a lot of time to get this right and explain it a short amount of time. Looking forward to part 3 and at stating you have another level how we can use linear regression analysis (ordinary least squares) with our 20 to 30 lab results to to find the line that most closely fits our data when working up a load at let’s say .3 grain increments vs MV. Luckily, we have online calculators, Excel, and OpenOffice that simplifies this for us and provides a nice chart to visualize it. Not that I’m trying to get you hooked on this stuff 🙂
Thanks, Joseph. I appreciate the encouragement. It was a tough process to write all this and make it cohesive and try to not go too overboard.
There are a lot of cool tools available to use today that make this much easier than it was just a few years ago. I think that will continue over the next several years. Data analysis and visualization is one of the places a TON of innovation is happening right now.
At the same time, I still think some pragmatism is appropriate here. Those of us with a heavier math background can can all go a bit overboard (me included), if we’re not careful. Ultimately, my experience is that many loads produce similar results. Unless you’re shooting Benchrest or ELR at the highest levels of competition, then the tiny differences may not worth chasing. That’s especially true if you’re making decisions based on insufficient data, but even once you have sufficient data, what I’ve found is there are typically a few loads that produce good results. So I’ve learned to just pick one, and go load it up and start practicing. The practice will do more for your ability to hit targets than trying to eek out that last bit of performance from a load.
I’ll actually touch more on that in the next post, but I couldn’t resist mentioning it here. It’s something I have to remind myself of, or I’ll look up and I’ve spent days testing loads and have a spreadsheet with 20 tabs of advanced analysis on the results. Can’t make that stuff up! 😉 It’s certainly hard for me not to go overboard – maybe not just here, but in life! Ha!
Thanks,
Cal
Can you recommend any reference that summarizes the development of how to obtain low standard deviation and extreme spread. There is so much info out there, some better than others. You got me excited with the last two posts on statistics and that is doing something. I don’t do math, in fact my 9th grade teacher gave me a d minus because she said I at least tried. Thank you for inspiring me and I’m sure others to new levels.
That’s a great question, Greg. And I’m glad to hear that I got you excited about this stuff. It really can be extremely helpful!
I racked my brain, and I can’t remember any references that I’d recommend. Maybe one of the other readers here knows of something and can share it. If nobody can point to anything online, maybe I’ll write something at some point. I think everyone would string me up if I did that before writing and publishing the 6.5 Creedmoor Match Ammo Field Test results, but I can see how something like that would be useful – especially if you could lay out the workflow in a visual way so guys could understand it and reference it at a glance.
Anyone know of a reference for load development that takes a more statistics-based approach than the traditional mix of small sample sizes and palm reading methods?
Thanks,
Cal
sounds like a opportunity Cal! I think even with modest sample sizes a disciplined approach with some simple techniques like randomizing, blind-ish, monitor and shoot on similar temp and wind days, cadenced shooting to keep temps close, break out deviations in x and y axis etc………may be a step in the direction. you can name the process this way!
Cal, tis “BIG SAMPLE” illustration of reliable extreme spread statistics is an excellent example of Thorough Testing v.s. limited testing. Andit illustrates behold “Garbage In – Garbage Out” mantra.
My Magnetospeed V3 crunches the data for me but ONLY gives me what I put in. More input = more reliable data.
That’s Eric. I’m with you. When I first read that Engleman study with the random subsets taken from a 50 round sample, I thought it was such a great way to think about this. It speaks pretty clearly and gives a good idea of the kind of variance we can see within the same exact batch of ammo, depending on how big your string is. It seems like I’ve read a thick stack of white papers on this kind of stuff, but that study kept sticking in my mind. It was pretty simple – but so helpful.
And I’m totally with you on the data only being as good as the samples you give it. The MagnetoSpeed or LabRadar will precisely calculate the stats for the bullets you shot over it … but it won’t tell you how good a prediction that is of what will happen in the future, and often that is a critical part of what would be helpful to know.
Also, it’s not those devices couldn’t calculate the expected range for a population based on a specific confidence interval, like 90% confidence or something. They just haven’t been programmed to do that. I think that’d be a super-useful feature. Adam’s online calculator does it. The math is well-defined. You just need to know the total # of shots, average, and SD of the sample, and you can predict the range for the average and SD for the population for a 90% confidence interval. That seems like that’d be a killer feature on a chronograph, and would make it much more useful. Maybe one of those manufacturers will read this. I actually bet that someone from those organizations (LabRadar and MagnetoSpeed and maybe even Ken Oehler) are reading this, and I wouldn’t be surprised if we don’t see a feature like that appear on a chronograph a year from now as a result of this series of articles. 😉
Thanks,
Cal
Cal, Great article, you obviously have put a lot of energy into your work, and I appreciate learning from it.
I want to pass on some info on how I have been working with ballistics, in relation to BC and ES. I am mostly in the 6mm and 6.5mm realm for precision at distance (1000yd max). Over the last few years, I have collected data from all weather conditions, in the pursuit of consistent loads for all seasons. I run a Magneto speed on all rifles (off rifle mount), and ran the magneto speed in conjunction with the Lab Radar for over a year (will share that in a bit).
My approach to precision, is to maintain the lowest ES possible, so that other adjustments (tuning, seating depth, etc.) will be more meaningful. I believe that low and consistent ES shows that the loading process is correct. When developing a load, I try to find the largest node to work in, and use “Quickload” to determine optimum barrel time. Once determined, I adjust the load to stay within that velocity (barrel time), regardless of atmospherics. I run my Magneto speed on all sessions except competitions. I also have tuners on all rifles.
As others have mentioned, BC’s are tweaked based on real world data, and kept for future use.
During sessions, I note and mark any anomaly cartridges (high or low velocity), and segregate them for future firings. Surprisingly, many of those cases repeat their performance.. I do modify my data to exclude anomalies, which is usually 4-8/100 in the first firing, reducing to 2-3/100 by the third firing. I have been able to hold an average 13ES in the 6.5 cartridges, and 6ES for the BR and BRA rounds. The data sample is thousands of rounds with four different rifles and several barrels, over a three year timeframe.
As to running the Magneto speed concurrent with the Lab Radar, I found the Lab Radar to read 4fps faster in overall average. Additionally, the Magneto speed drops 2-3 shots/1000, the LR drops 3-4/100. I recently put a remote trigger on the LR, and it seems to be helping..
Thanks again for all your hard work, keep it up!
Dennis
Wow, Dennis! That is some impressive work. I think that is the lowest ES I’ve ever heard of, but I do know a lot of shooters that believe the 6 BRA is likely the easiest cartridge to load for. One of my friends refers to it as “Reloading for Dummies” … but he still isn’t getting down to a 6 fps ES!
Your comment about segregating brass that seems to fire significantly slower or faster than the majority reminds me of a similar conversation I had with a serious Benchrest shooter. He echoed those same sentiments that if a round didn’t go into a group with the rest of the shots, it would likely repeat that on the next loading. He was convinced that it wasn’t necessarily the powder charge, primer, etc., but something to do with the brass. He even said there were times that the brass had identical external dimensions, weight, neck tension, and other specs as the other cases, but for whatever reason, if it didn’t go into the group … it likely wouldn’t ever go into the group. I don’t shoot Benchrest and haven’t done my own testing related to that, but it’s a very interesting concept and it at least seems like your experience roughly corroborates that theory.
I am actually planning to build a fully enclosed, 100-yard underground shooting tunnel at my home, and once that is in place I hope to be able to test things like that. It will be at least a year before that is up and functioning, but I am already dreaming of a day when I can do experiments like that!
I appreciate you sharing your process and experience, and for the kind words about my content.
Thanks,
Cal
Hi Cal. I just read and commented on Part 1 of this article series. In that comment I voted for ES as the data point I want to minimize in load development. Now I’ve read this Part 2 where you compare and contrast SD vs ES. While I agree with your explanations of the statistical theory (very nice job on that), I still think that for me, Minimum ES will be my loading goal. Why? Because my goal in loading is to have the exact same muzzle velocity each shot. ES tells me that directly. (Assuming a reasonable sample size, and I agree a sample size of 20 is a good balance between cost of testing and confidence in results.)
If my ES in a 20-shot sample is more than 10 fps, then there is something inconsistent in my loading. Some variable is awry and not being controlled properly. An ES more than 10 tells me to go back and find where the variation crept in and fix it. Did I change power lot numbers in the middle of a loading session? Is one of my dies wearing or came out of adjustment? I need to find the reason and correct it, and a high ES is my warning flag to do so.
I believe that with highly consistent loading processes,I will enjoy a low ES even over larger sample sizes. If the process is controlled tightly enough, every single shot should leave the muzzle at the same velocity…an ES of zero, even over 100 shots. I can’t reach that ideal minimum because I can’t spend enough for the precision measuring equipment, powder scales mostly, and I can’t afford the time to let the barrel cool to the same exact temperature each shot. ButI can get under 10 fps consistently.
Steve, if you can get an ES under 10 fps over large sample sizes (20 or more consecutive shots) that would be awesome! I think you will find that is harder than you think, and maybe not even in the realm of possibility. That would put your SD around 1.6 fps, which I’ve certainly never heard of over a 20-shot string – even for those clinically OCD guys that have a ton of experience and all the high-end equipment. I’ve never got even close to that. But, if you read all this – at least you’re walking away with an educated decision.
Best of luck to you!
Cal
Question for the experienced load developers. It would seem to me that the variation in MV would tend to result in variation in shot placement in the Y-axis (vertical) and not (or much less) in the horizontal or X-axis. Is this a reasonable assumption? If so, would not the evaluation of loads and associated MV data be better described by the Y-axis component of the shot spread for each shot? I only ever see folks looking at group size but a shot .25″ high and 1″ right seems like the .25″ component is more telling? I guess we’re talking about a sensitivity analysis of MV to X and Y deviations. If this logic plays out this would make for a much cleaner dataset of MV vs. Y-axis deviations.
Hey, Paul. I can see your logic and there may be some portion of vertical dispersion related to muzzle velocity, but at short range there is radial dispersion in x and y due to a number of mechanical factors. Let’s think about the variation in the X axis. There might be a slight component to horizontal dispersion at short range due to wind, but there is still dispersion in the X axis even if you are shooting in a fully enclosed tunnel. Likewise, there would still be dispersion in the Y axis even if our muzzle velocity was EXACTLY the same for each shot. At short range, I think of dispersion as mostly due to mechanical precision, but the dispersion in the Y direction does grow with distance if there is muzzle velocity variation. That’s why we obsess over muzzle velocity consistency in the long range world, but I simply don’t think you see it on a target at 100 yards like you might be thinking.
Someone else can chime in if they feel different or know of any research showing something different.
Hope that’s helpful!
Thanks,
Cal
Great as usually!
Thank you.
Thanks!
Just some technical comments that may help. The sample standard deviation (i.e., the formula for “s”, which is an estimator of “sigma” – the unknown true standard deviation) is biased. If the muzzle velocities are normally distributed, then a formula for correcting the bias exists. The amount of correction depends on how many shots are fired and measured. As this number increases the bias tends to zero. In the quality control world the bias correction factor is known as “c4” and is tabulated (a formula for c4 also exists and can be computed in Excel).
As an example, with 5 shots dividing the computed s by 0.94 makes it an unbiased estimate. So as noted earlier, computed s values should be corrected “up”.
ES is also known as the “Range”. If muzzle velocities are normally distributed, then the Relative Range random variable is defined as the Range divided by the true standard deviation (unknown). The Relative Range random variable has been analyzed numerically when the random variable of interest in normal (i.e. muzzle velocity), and it is possible to get an unbiased estimate of sigma from the Range (ES) using tabulate constants referred to as “d2” in the QC world. It’s a slightly less efficient estimator than the corrected sample std dev, but it’s much simpler to compute.
General recommendations in QC chart construction is to use Range to estimate sigma for lower data numbers (approx less than 10), and use sample standard deviation for more data.
I realize this is maybe splitting hairs for some and also technically boring for others. I hope it does add to the comparison of ES and sample standard deviation.
While that is probably more technical than a lot of my readers would appreciate – it’s very interesting to me! 😉 I guess that is the more technical reason that so many of us say you should never rely on an SD if there weren’t at least 10 shots in the string. I seriously ignore the SD for any strings I fire that aren’t at least 10 shots, and if I’m really trying to characterize the variation for a large sample or new load – even 10 is low. It’s funny how we as shooters can forget some of the basics of statistics when we get behind a gun!
Thanks for taking the time to type it all out. I really did think it was interesting, and I actually hadn’t heard or read a good bit of that.
Thanks,
Cal